AI & MILITARY PROCUREMENT: WHAT COMPUTERS STILL CAN’T DO
9 min read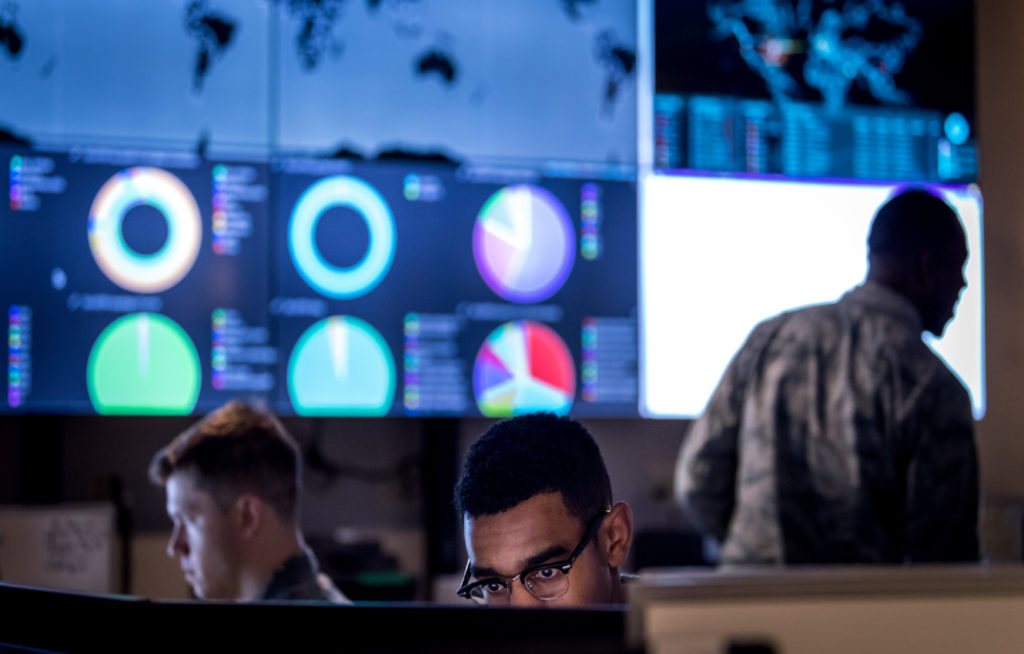
MAAIKE VERBRUGGEN
Not all artificial intelligence (AI) is made equal. A wide range of different techniques and applications fall under the term “AI.” Some of these techniques and applications work really well, such as image recognition. But other AI applications, especially those focused on prediction, have fallen short of expectations.
Fielding AI before it is ready could lead to complacency amongst soldiers, inaccurate predictions, and strategic manipulation by users to game the system. Military procurement officers need to learn basic AI literacy to become smart buyers and ensure that AI is not fielded prematurely.
Manage Expectations of Artificial Intelligence
AI has improved significantly over the past 20 years, but there are still clear limits to what algorithms can do. AI is much better equipped at tasks related to categorization and classification than judgement or prediction. Princeton Associate Professor Arvind Narayanan refers to programs sold in the latter category as “AI snake oil.” AI is especially strong at tasks like image recognition, translation, and generating new content (e.g., deepfakes or artificially generated text such as Generative Pretrained Transformer-2 (GPT-2)_. These are tasks that are narrow, with a clearly defined purpose, and little ambiguity about the correctness of the outcome. AI is best-suited for such applications.
AI struggles more with tasks related to automated judgment, such as spam filtering and hate speech detection. These tasks involve a subjective verdict, and there will always be disagreement about the correctness of the decision. Most difficult is the task of prediction, especially for social outcomes. Narayanan claims that AI currently performs little better than linear regression. For example, Julia Dressel and Hany Farid have shown that software used to predict recidivism does not perform better than people without criminal justice expertise. Additionally, AI will perform worse if there is limited data, or if the data it is trained on and data from the real world are not similar. Moreover, it is still very difficult to integrate prior knowledge into deep learning models.
So far, AI has not yet lived up to the hype. A 2019 MIT survey of over 2,500 executives showed that 65 percent of the companies that made investments in AI have not yet seen any value gained from it in the past three years. Prof. Erik Brynjolfsson calls this the “productivity paradox of AI.” A similar dynamic is playing out with fully autonomous cars, that were predicted to drive on the streets years ago. That does not mean that no AI works, or that AI will never work. AI is great for many applications, but it’s just not a tool that can fix every problem.
Policymakers, engineers, and analysts should develop more realistic expectations of AI. If not, the result could be another “AI winter.” AI winters previously occurred in the 1970s and 1990s, as research funding dried up when high expectations were not met. The technologies of concern are not the ones that clearly don’t work, but those that give a false illusion of being capable. This is hard to judge. It is much less obvious whether a predictive program works than a tank does, and the outcome of the assessment whether software works is much more ambiguous. Moreover, there are no formal methods yet to verify and validate autonomous systems, making it even more difficult to assess whether programs function as prescribed. This will make procurement and deployment especially challenging.
Risks of Fielding Artificial Intelligence Prematurely
Fielding AI applications prematurely poses many risks for militaries, including complacency, inaccuracy, and strategic manipulation.
The first risk is that AI might provide a false sense of security and lead to complacency. As machines receive more responsibility, human involvement will take the form of a more supervisory level of control. If machines underperformed or made mistakes, this could go undetected due to reduced human engagement. One example concerns emotion detection or intent-recognition software. The European Union is currently developing AI-based lie detectors for use in border control. The problem is that emotion detection AI is based on outdated work in psychology, which suggests there are six elementary types of emotions, that all people across the globe express similarly. But this view is falling out of favor, with the largest meta-study to date showing that the expression of emotions varies across culture, people, and even situations. The same facial expression can be the result of different emotions and requires context to interpret. The premise is thus flawed. Experts have extensively critiqued the accuracy of emotion recognition software, especially when used on people of color. Of course, this is a problem for the people who might be denied entry to a country or falsely detained. But users are also at risk. If they believe the software works as promised, they could lower their guard, and assess situations less critically than they would otherwise. Threats might fly under the radar.
The second risk is that machines could make incorrect recommendations. One example is the use of AI for military recruitment. The United States is experimenting with a program that uses AI to assesses whether candidates would be suitable for U.S. Marine Corps special operations. As with emotion recognition, the scientific foundation for this is shaky. But the features that the machine identifies do not correspond with the actual properties it wants to measure. The problem is that machines do not always have access to the actual properties they want to evaluate, and often only evaluate an intermediate layer of features that influences the assessment. For example, standardized testing aims to measure students’ level of learning, but in practice measures many other variables as well, such as socio-economic status. Similar civilian recruitment software has been tried, with limited success — in one case, the algorithm found that being named Jared and having played lacrosse were key job performance indicators. Clearly, these qualities themselves do not say anything about job performance and are likely a proxy indicator for gender and socio-economic status. Using such systems risks excluding strong candidates from the armed forces, especially if they are from underrepresented groups.
The third risk is that soldiers could engage in suboptimal behavior for positive machine approval. Many AI applications currently under development are not meant to be used upon the enemy, but to manage the military workforce. Examples include the Integrated Visual Augmentation System of the U.S. military, which trains soldiers using augmented and virtual reality. It tracks soldiers’ eye and hand movements as well as their voice, and these biometrics — combined with behavioral data — are designed to “identify weak spots in individuals.” Another example is the project of the U.S. Defense Security Service to detect employees who “have betrayed their trust” and predict who might do so in the future, and thereby violate their security clearance.
AI experts have identified a process called strategic classification, in which people alter their behavior in order to get a better classification by the machine. This is not necessarily harmful when, for instance, students work to increase their grades to improve their chances of college admission. However, often people alter their behavior in ways that that the machine approves of, but that does not actually produce a positive effect (e.g., when people open multiple credit lines in order to improve their credit scores). As soldiers are monitored more, they will be evaluated and judged according to metrics developed by AI. But evaluating soldier performance takes place in a complex environment, requires context, and is subjective. AI struggles with all three of these characteristics, raising questions about its accuracy. Consequently, soldiers might alter their behavior to meet potentially inadequate metrics, which could be suboptimal from an operational, safety, legal, and/or ethical point of view.
Why Artificial Intelligence Might Be Fielded Anyway?
The problems laid out here cannot be easily waved away. Incorporating AI into military procurement will prove especially challenging. There are several risks factors at play here particular to AI.
First, the hype surrounding AI is not helping. The positive narratives in the media help shape the idea of AI as already highly successful. The general public expects much more rapid progress than experts do. A 2019 public opinion poll showed that the U.S. public thinks there is a 54 percent likelihood of developing high-level machine intelligence within 10 years, and a 2018 global opinion poll revealed that the general public predicts a 50 percent probability of high-level machine intelligence in 2039. Robotics experts, on the other hand, predict a 50 percent probability only in 2065, and machine learning experts in 2061. The public thus overestimates the progress made by AI. Of course, the general public does not procure weapon systems, but it does illustrate how important it is that decision-makers in military procurement have a solid understanding of how AI works.
Second, as the geopolitical climate has become more tense, talk of a “AI arms race” has increased. Concerned that they are falling behind their rivals, countries could feel the pressure to deploy AI as soon as possible, potentially before the technology had fully matured, and before it has been tested and evaluated extensively.
Third, Silicon Valley has a tendency to overpromise, which influences the general conception of AI. It is not uncommon to “fake it till you make it” — promise an interesting and appealing new technology in order to acquire funding, long before the technology has actually been developed fully. This leads to overhype and false promises. If development turns out to be less successful than planned, it is not unheard of to market products as autonomous when they are actually operated by humans, a practice called “fauxtomation.”
Another consequence is that instead of improving the machine to become capable of operating in the real world, some are tempted to alter the real world to allow the machine to operate. An example is the calls from autonomous vehicle advocates to alter existing infrastructure and create spaces not used by normal cars, bikes, and pedestrians, so the autonomous cars can drive in more predictable environments. This creates an illusion that technologies work when they actually don’t, or only under simplified conditions.
Guidelines for Military Procurement of AI
Currently AI excels at narrow, tailored applications, but it struggles with real-world complexity, especially when judging and predicting social contexts. Deploying the technology before it is ready could lead to a false sense of security, incorrect machine recommendations, and suboptimal military behavior. This is not to suggest that AI is completely defunct. A lot of AI is very promising. However, there are still some serious flaws in certain applications of AI. Experts should carefully consider which applications are best-suited for AI, and policymakers should not be hasty in deploying AI before it is mature.
To avoid these risks, military procurement should follow three guidelines.
First, do not develop AI for the sake of AI. AI has many highly beneficial uses, but it is not a panacea. It is important to first clearly identify a problem, and only then consider when AI can be a potential solution for this problem. AI should be used for a specific purpose, and not be used for its own sake. Promising use-cases of AI include optimizing the energy usage of data centers, electromagnetic spectrum management, translating unfamiliar languages in the field, and detecting mines.
Second, militaries need to recruit AI experts who have an in-depth understanding of the technology in order to act as smart buyers. This can take the form of external recruitment, internal education, incentive programs for staff to acquire technical skills, or even a complete reform of the personnel process. The lack of technical knowledge has been well-documented.
This is a serious problem, as military bureaucracies do not have the right technical knowledge in-house due to a severe shortage of AI talent. Moreover, the people who actually have this technical knowledge are often not rewarded for their skillsets, find themselves isolated from the dominant organizational culture, and face a lack of recognition in their career progression. It is unnecessary (and unrealistic) for everyone to become AI engineers, but the defense workforce needs to reach a higher level of AI literacy (as Michael Horowitz and Lauren Kahn call it) to ensure decision-makers understand the key principles and potential limitations of AI. This knowledge is necessary even when not developing the systems internally, in order to identify potential problems, and consider whether a specific application of AI is the right tool for the job.
Finally, money should be invested in developing alternative techniques for the verification and validation of autonomous systems.
Through thoughtful, critical, and informed procurement, militaries can avoid fielding AI prematurely and taking unnecessary risks. AI can be very useful, but it is just a tool to achieve larger objectives.